Laura Smith
Hello! I'm a final-year PhD student in CS at UC Berkeley advised by Sergey Levine. I spent an amazing summer in 2024 working with the Google DeepMind Robotics team. I'm currently interning at Physical Intelligence.
I work on enabling robots to interact with and learn in the real world, so as to acquire human-like abilities.
My early PhD was supported in part by the NSF Graduate Research Fellowship, and I am thankful to be supported now by a Google PhD Fellowship.
I am graduating in May 2025 and seeking full-time opportunities in industry research labs. Please connect via email if I might be a good fit for your team!
CV
Github
G. Scholar
Twitter
smithlaura at berkeley dot edu
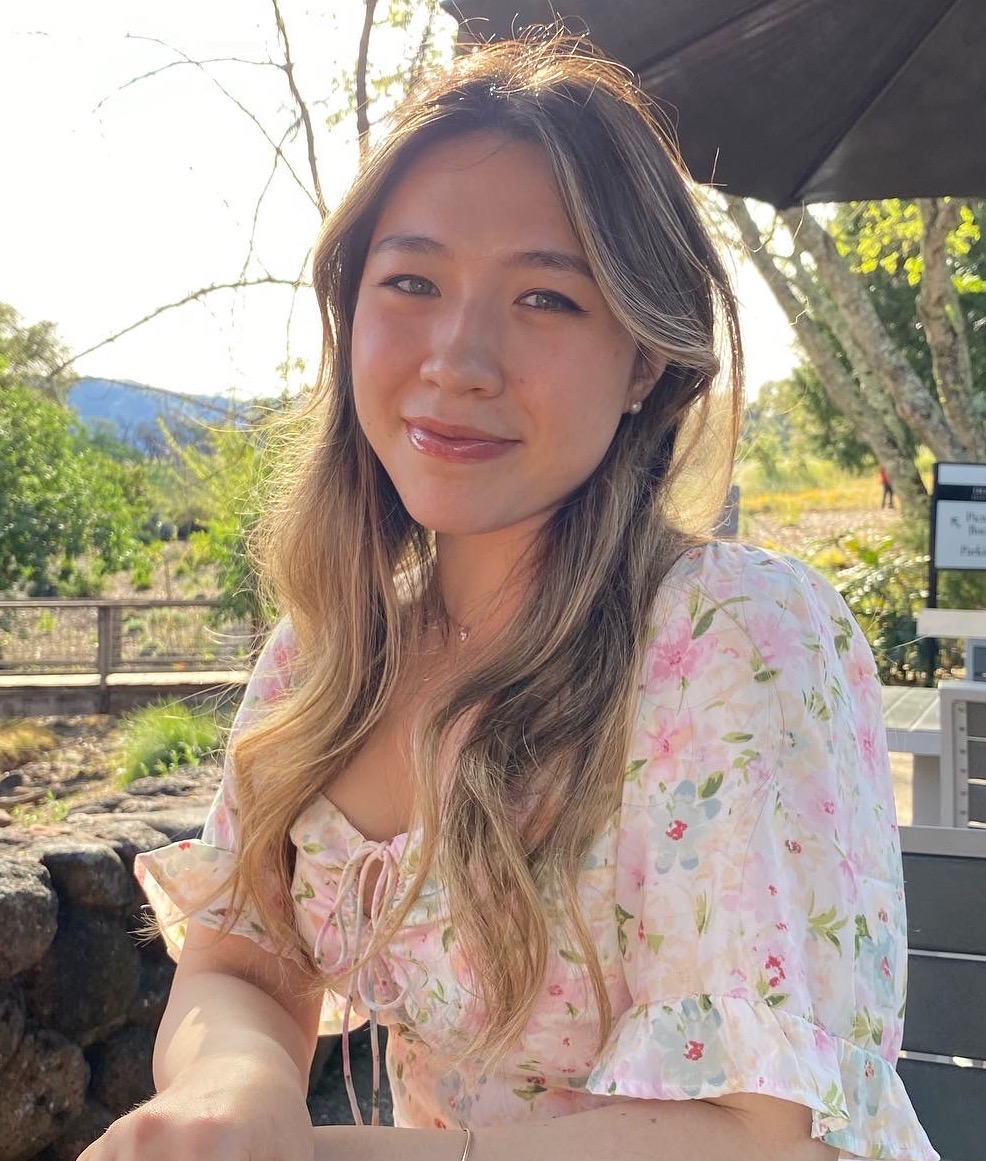
The following are most representative of my personal work.
For a complete list of publications, please see my Google Scholar profile.
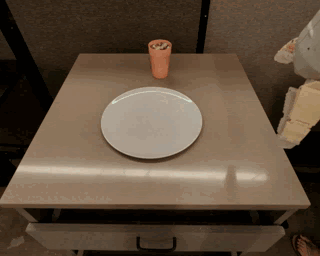
STEER: Flexible Robotic Manipulation via Dense Language Grounding
Laura Smith, Alex Irpan, Montserrat Gonzalez Arenas, Sean Kirmani, Dmitry Kalashnikov, Dhruv Shah, Ted Xiao
in submission
Webpage •
PDF
Grow Your Limits: Continuous Improvement with Real-World RL for Robotic Locomotion
Laura Smith*, Yunhao Cao*, Sergey Levine
ICRA 2024
Webpage •
PDF
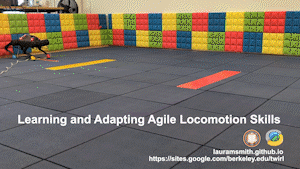
Learning and Adapting Agile Locomotion Skills by Transferring Experience
Laura Smith, J. Chase Kew, Tianyu Li, Linda Luu, Xue Bin Peng, Sehoon Ha, Jie Tan, Sergey Levine
RSS 2023
Webpage •
PDF
RLPD: Efficient Online Reinforcement Learning with Offline Data
Philip J. Ball*, Laura Smith*, Ilya Kostrikov*, Sergey Levine
ICML 2023.
PDF •
Code
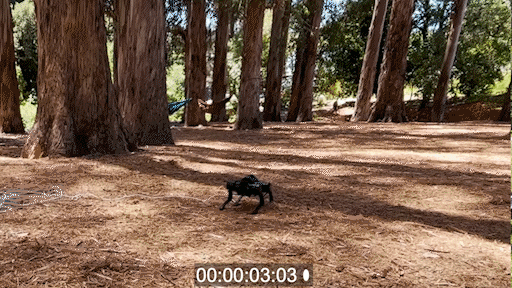
A Walk in the Park: Learning to Walk in 20 Minutes With Model-Free Reinforcement Learning
Laura Smith*, Ilya Kostrikov*, Sergey Levine
RSS Demo Track, 2023.
Webpage •
PDF •
Code
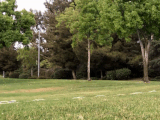
Legged Robots that Keep on Learning: Fine-Tuning Locomotion Policies in the Real World
Laura Smith, J. Chase Kew, Xue Bin Peng, Sehoon Ha, Jie Tan, Sergey Levine
ICRA 2022.
Webpage •
PDF •
Code
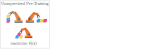
PEBBLE: Feedback-Efficient Interactive Reinforcement Learning via Relabeling Experience and Unsupervised Pre-Training
Kimin Lee*, Laura Smith*, Pieter Abbeel
ICML 2021, Long Oral Presentation.
Webpage •
PDF •
Code
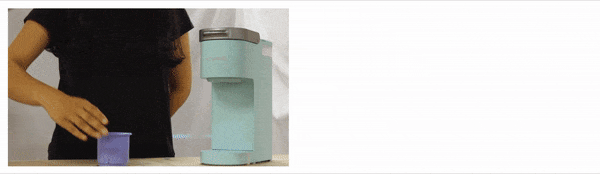
AVID: Learning Multi-Stage Tasks via Pixel-Level Translation of Human Videos
Laura Smith, Nikita Dhawan, Marvin Zhang, Pieter Abbeel, Sergey Levine
RSS 2020.
Webpage •
PDF •
BAIR Blog
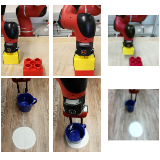
SOLAR: Deep Structured Latent Representations for Model-Based Reinforcement Learning
Marvin Zhang*, Sharad Vikram*, Laura Smith, Pieter Abbeel, Matthew Johnson, Sergey Levine
ICML 2019.
Webpage •
PDF •
Code •
BAIR Blog
2022
Co-Organizer, CoRL Workshop on Learning to Adapt and Improve in the Real World2022+
Reviewer, CoRL, RA-L, ICRA, NeurIPS, IROS, ICLR.2020+
Co-Organizer, Berkeley AI Research Mentoring Program2018-2020
Co-Organizer, Robot Learning Lab Outreach2018
Guest Lecture at UC Berkeley CS10: The Beauty and Joy of Computing (video)